The Spatio-Temporal Modeling of Urban Growth Using Remote Sensing and Intelligent Algorithms, Case of Mahabad, Iran
Abstract
The simulation of urban growth can be considered as a useful way for analyzing the complex process of urban physical evolution. The aim of this study is to model and simulate the complex patterns of land use change by utilizing remote sensing and artificial intelligence techniques in the fast growing city of Mahabad, north-west of Iran which encountered with several environmental subsequences. The key subject is how to allocate optimized weight into effective parameters upon urban growth and subsequently achieving an improved simulation. Artificial Neural Networks (ANN) algorithm was used to allocate the weight via an iteration approach. In this way, weight allocation was carried out by the ANN training accomplishing through time-series satellite images representing urban growth process. Cellular Automata (CA) was used as the principal motor of the model and then ANN applied to find suitable scale of parameters and relations between potential factors affecting urban growth. The general accuracy of the suggested model and obtained Fuzzy Kappa Coefficient confirms achieving better results than classic CA models in simulating nonlinear urban evolution process.
Downloads
References
Alkheder, SH., 2006. Urban growth modeling with artificial intelligence techniques. PhD Dissertation, Purdue University, USA.
Allen, PM. 1997. Cities and regions as self-organizing systems: models of complexity, Gordon and Breach Science, Amsterdam.
Batty, M. 2005. Cities and complexity: understanding cities with cellular automata, agent-based models, and fractals, The MIT Press, Cambridge.
Batty, M., 1998. Urban evolution on the desktop: simulation with the use of extended cellular automata, Environment and Planning A., 30(11): 1943 – 1967.
Batty, M., and Longley, P., 1994. Fractal cities: geometry of form and function, Academic Press, London.
Batty, M., Xie, Y., and Sun, Z., 1999. Modelling urban dynamics through GIS-based cellular automata. Computers, Environment and Urban Systems, 23: 205–233.
Bockstael, NR., Costanza, I., Strand, W., Boyton, K., Bell, S., and L. Wagner., 1995. Ecological economics modeling and evaluation of ecosystems. Ecological Economics: 14: 143–159.
Cecchini, A., Rizzi, P., 2001. The reasons why cellular automata are a useful tool in the working-kit for the new millennium urban planner in governing the territory. In: CUPUM 2001 Proceeding, Honolulu.
Erfu, D., and Shaohong, W., 2005. Modeling change-pattern-value dynamics on land use: an integrated GIS and artificial neural networks approach, Journal of Environmental Management, 36(2): 1–17.
Hagen, A., 2003. Fuzzy set approach to assessing similarity of categorical maps. International Journal of Geographical Information Science, 17(3): 235-249.
He, C., Okada N., Zhang Q., Shi P. and Li J., 2008. Modeling dynamic urban expansion processes incorporating a potential model with cellular automata, landscape and urban planning, 86: 79-91.
Hegde, S., 2003. Modeling land cover change: A fuzzy approach, International Institute for Geo-Information Science and earth Observation Enscheda, Netherlands.
Itami, R., 1994. Simulation of spatial dynamics: cellular automata theory. Journal of Landscape and Urban Planning. 30: 27–47.
Kavzoglu, T., and Mather, PM., 2003. The use of back propagation artificial neural networks in land cover classification. International Journal of Remote Sensing, 24: 4097–4938.
Li, X., and Yeh, AGO., 2002. Neural network-based cellular automata for simulating multiple land use changes using GIS. International Journal of Geographical Information Science, 16(4): 323–343.
Li, X., and Yeh., AGO., 2000. Modeling sustainable urban growth by the integration of constrained cellular automata and GIS. International Journal of Geographical Information Science, 14:131–152.
Li, X., Yang, Q., and Liu, X., 2008. Discovering and evaluating urban signatures for simulating compact development using cellular automata. Journal of Landscape and Urban Planning, 86: 177–186.
Li, X., and Yeh, AGO., 2004. Data mining of cellular automata’s transition rules. International Journal of Geographical Information Science, 18(8): 723-744.
Li, L. Sato, Y. and Zhu, H., 2003. Simulating spatial urban expansion based on a physical process. Journal of Landscape and Urban Planning, 64: 1, 67-76.
Mahini, AR., and Kamyab, A., 2010. Application of ANN in urban development. Journal of Iranian Human Geography, 76: 49-67.
Maithani, S., 2009. A neural network based urban growth model of an Indian city, Journal of Indian Society of Remote Sensing, 37: 363–376.
Onishi, T. and Braimoh, AK., 2007. Geostatistical techniques for incorporating spatial correlation into land use change models, International Journal of Applied Earth Observation and Geoinformation, 9: 438-443.
Pontius RG., Shusas JE., and McEachern, M., 2004. Detecting important categorical land changes while accounting for persistence. Agriculture, Ecosystems and Environment, 101: 251-268.
Portugali, J., Benenson, I., and Omer, I., 1997. Spatial cognitive dissonance and socio-spatial emergence in a self-organizing city. Environment and Planning B, 24: 263– 285.
Santé, I., Garcia, AM., Miranda, D., and Crecente, R., 2010. Cellular automata models for the simulation of real-world urban processes: a review and analysis. Journal of Landscape and Urban Planning, 96: 108-122.
Straatman, B., White, R. and Engelen, G., 2004. Towards an automatic calibration procedure for constrained cellular automata. Computers, Environment and Urban Systems, 28: 23-31.
Rumelhart, DE., McClelland, JL., and the PDP Research Group, 1986. Parallel Distributed Processing: Explorations in the Microstructure of Cognition. MIT Press, Cambridge.
Torrens, PM. and O'Sullivan, D., 2001. Cellular automata and urban simulation: where do we go from here? Environment and Planning B, 28: 163-168.
Turner, BL., 1994. Local faces, global flows: the role of land use and land cover in global environmental change, Land Degradation and Development, 5: 71-78.
Wolfram, S., 1984. Communications in Mathematical Physics, 96: 15-57.
Wolfram, S., 2002. A new kind of science. Wolfram Media, Inc.
Wu, F., 2002. Calibration of stochastic cellular automata: the application to rural-urban land conversions. International Journal of Geographical Information Science, 16: 795–818.
Wu, F., and Webster, CJ., 1998. Simulation of land growth through the integration of cellular automata and multi-criteria evaluation, Environment and Planning B, 25: 103–111.
Yeh, AGO. and Li, X., 2002. A cellular automata model to simulate development density for urban planning. Environment and Planning B, 29: 431-450.
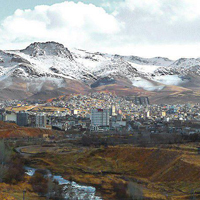
Copyright (c) 2014 Tema. Journal of Land Use, Mobility and Environment

This work is licensed under a Creative Commons Attribution 4.0 International License.
Authors who publish in this journal agree to the following:
1. Authors retain the rights to their work and give in to the journal the right of first publication of the work simultaneously licensed under a Creative Commons License - Attribution that allows others to share the work indicating the authorship and the initial publication in this journal.
2. Authors can adhere to other agreements of non-exclusive license for the distribution of the published version of the work (ex. To deposit it in an institutional repository or to publish it in a monography), provided to indicate that the document was first published in this journal.
3. Authors can distribute their work online (ex. In institutional repositories or in their website) prior to and during the submission process, as it can lead to productive exchanges and it can increase the quotations of the published work (See The Effect of Open Access)