Formal Ontologies and Uncertainty. In Geographical Knowledge
Abstract
Formal ontologies have proved to be a very useful tool to manage interoperability among data, systems and knowledge. In this paper we will show how formal ontologies can evolve from a crisp, deterministic framework (ontologies of hard knowledge) to new probabilistic, fuzzy or possibilistic frameworks (ontologies of soft knowledge). This can considerably enlarge the application potential of formal ontologies in geographic analysis and planning, where soft knowledge is intrinsically linked to the complexity of the phenomena under study. The paper briefly presents these new uncertainty-based formal ontologies. It then highlights how ontologies are formal tools to define both concepts and relations among concepts. An example from the domain of urban geography finally shows how the cause-to-effect relation between household preferences and urban sprawl can be encoded within a crisp, a probabilistic and a possibilistic ontology, respectively. The ontology formalism will also determine the kind of reasoning that can be developed from available knowledge. Uncertain ontologies can be seen as the preliminary phase of more complex uncertainty-based models. The advantages of moving to uncertainty-based models is evident: whether it is in the analysis of geographic space or in decision support for planning, reasoning on geographic space is almost always reasoning with uncertain knowledge of geographic phenomena.Downloads
References
Abulaish M., Dey L. (2003) Ontology Based Fuzzy Deductive System to Handle Imprecise Knowledge. In Proceedings of the 4th International Conference on Intelligent Technologies, pp. 271-278.
Bakillah M., Mostafavi M. A. (2011) A Fuzzy Logic Semantic Mapping Approach for Fuzzy Geospatial Ontologies. SEMAPRO 2011 The Fifth International Conference on Advances in Semantic Processing, IARIA, pp. 1-8.
Ban H., Alhqvist O. (2009) Representing and Negotiating Uncertain Geospatial Concepts – Where Are the Exurban Areas? Computers, Environment and Urban Systems, issue 33, pp. 233-246.
Bobillo F., Straccia U. (2010) Fuzzy Ontology Representation using OWL 2. arXiv:1009.3391v3 [cs.LO] 4 Nov 2010, pp. 1-32.
Bouchon-Meunier B. (1994) La logique Floue. Paris, Presses Universitaires de France, 128 p.
Caglioni M., Rabino G. (2012) Ontologies in multi-agent systems for building design the case of risk management inside a stadium. In Billen R., Caglioni M., Marina O., Rabino G., San José R. (Eds.) 3D Issues in Urban and Environmental Systems. Società Editrice Esculapio, Bologna, pp. 111-118.
Caglioni M., Rabino G. (2007) Theoretical approach to urban ontology: a contribution from urban system analysis. Published in Teller J., Lee J. R., Roussey C. (Eds.) Ontologies for Urban Development of Studies in Computational Intelligence, Volume 61, Springer Berlin / Heidelberg, pp. 109-119.
Costa P.C.G., Laskey K.B., Laskey K.J. (2008) PR-OWL: A Bayesian Ontology Language for the Semantic Web. LNAI 5327, Springer-Verlag Berlin Heidelberg, pp. 88-107.
De Runz C. (2008) Imperfection, temps et espace: modélisation, analyse et visualisation dans un SIG archéologique. Thèse de doctorat de l’Université de Reims Champagne-Ardenne (URCA), 230 p.
Dempster A.P. (1968) A generalization of Bayesian inference. Royal Statistical Society Journal, 30(2) pp. 205–247.
Dempster A.P., Laird N.M., Rubin D.B. (1977) Maximum likelihood from incomplete data via the EM algorithm (with discussion). J R Stat Soc Series B (Statistical Methodology), n. 39, pp. 1–38.
Ding Z., Peng Y. (2004) A Probabilistic Extension to Ontology Language OWL. Proceedings of the 37th Hawaii International Conference on System Sciences, pp. 1-10.
Dubois D., Prade H. (1985) Théorie des Possibilités, Paris, Masson
Dubois D., Lang J., Prade H. (1994) Possibilistic logic. In Handbook of logic in Artificial Intelligence and Logic Programming, Volume 3, Oxford University Press, pp. 439–513.
Fisher P., Comber A., Wadsworth R. (2006) Approaches to Uncertainty in Spatial Data. In R. Devillers and R. Jeansoulin (Eds.) Fundamentals of Spatial Data Quality, pp. 43–59.
Fonseca F., Camara G., Monteiro A.M. (2005) A Framework for Measuring the Interoperability of Geo-Ontologies. In Spatial Cognition and Computation, vol. 6, issue 4, 2005, pp. 307-329.
Fusco G. (2004) Looking for Sustainable Urban Mobility through Bayesian Networks. Cybergeo, http://cybergeo.revues.org/2777
Fusco G. (2012) Démarche géo-prospective et modélisation causale probabiliste. Cybergéo, http://cybergeo.revues.org/25423
Ghorbel H., Bahri A., Bouaziz R. (2008) A Framework for Fuzzy Ontology Models. Proceedings of Journées Francophones sur les Ontologies JFO’2008, Lyon, France, pp. 21-30.
Goodchild M.F. (2007) Citizens as Voluntary Sensors: Spatial Data Infrastructure in the World of Web 2.0. International Journal of Spatial Data Infrastructures Research, 2, pp. 24–32.
Loiseau Y., Boughanem M., Prade H. (2006) Evaluation of Term-based Queries using Possibilistic Ontologies. Soft Computing in Web Information Retrieval, Studies in Fuzziness and Soft Computing, Volume 197, pp 135-160.
Murgante B., Scorza F. (2011) Ontology and Spatial Planning. ICCSA 2011, Part II, LNCS 6783, pp. 255–264.
Qi G., Ji Q., Pan J.Z., Du J. (2010) PossDL - A Possibilistic DL Reasoner for Uncertainty Reasoning and Inconsistency Handling. In L. Aroyo et al. (Eds.), ESWC 2010, LNCS 6089, pp. 416–420.
Shafer G. (1976) A Mathematical Theory of Evidence. Princeton University Press, Princeton, N.J., USA.
Straccia U. (2006) A Fuzzy Description Logic for the semantic Web. Proceedings in Capturing Intelligence: Fuzzy logic and the semantic Web, Elie Sanchez ed., Elsevier.
Studer R., Benjamins V., Fensel D. (1998). Knowledge Engineering: Principles and Methods. Data and Knowledge Engineering, 25(1-2), pp. 161-197.
Walker W. et al. (2003), Defining Uncertainty. A Conceptual Basis for Uncertainty Management in Model-Based Decision Support. Integrated Assessment, vol. 4, no 1, pp. 5-17
Wang Y., Liu W., Bell D. (2007) Combining Uncertain Outputs from Multiple Ontology Matches, in H. Prade and V.S. Subrahmian (Eds.), SUM 2007, LNAI 4772, Springer, pp. 201-214
Zadeh L.A. (1965) Fuzzy sets. Information and Control, vol. 8, no 3, pp. 338-353.
Zadeh L.A. (1978) Fuzzy sets as a basis for a theory of possibility. Fuzzy Sets and Systems, 1, pp. 3–28.
Zhu M., Gao Z., Pan J.Z., Zhao Y., Xu Y., Quan Z. (2013) Ontology Learning from Incomplete Semantic Web Data by BelNet. In Proceedings of the IEEE International Conference on Tools with Artificial Intelligence (ICTAI 2013), pp. 761-768.
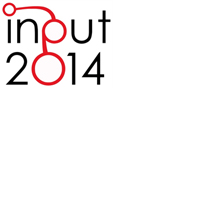
Copyright (c) 2014 Tema. Journal of Land Use, Mobility and Environment

This work is licensed under a Creative Commons Attribution 4.0 International License.
Authors who publish in this journal agree to the following:
1. Authors retain the rights to their work and give in to the journal the right of first publication of the work simultaneously licensed under a Creative Commons License - Attribution that allows others to share the work indicating the authorship and the initial publication in this journal.
2. Authors can adhere to other agreements of non-exclusive license for the distribution of the published version of the work (ex. To deposit it in an institutional repository or to publish it in a monography), provided to indicate that the document was first published in this journal.
3. Authors can distribute their work online (ex. In institutional repositories or in their website) prior to and during the submission process, as it can lead to productive exchanges and it can increase the quotations of the published work (See The Effect of Open Access)